Ariel Procaccia
Harvard University
I am the Alfred and Rebecca Lin Professor of Computer Science at Harvard University. I am a member of the EconCS Group; I am also affiliated with the Center for Research on Computation and Society, the Harvard Data Science Initiative, the Ash Center for Democratic Governance and Innovation, the Institute for Quantitative Social Science and the Center of Mathematical Sciences and Applications.
I work on a broad and dynamic set of problems related to AI, algorithms, economics, and society. I am especially excited about projects that involve both interesting theory and direct applications; examples include the websites Spliddit and Panelot, as well as recent collaborations with nonprofit organizations such as Refugees.AI, 412 Food Rescue, and the Sortition Foundation.
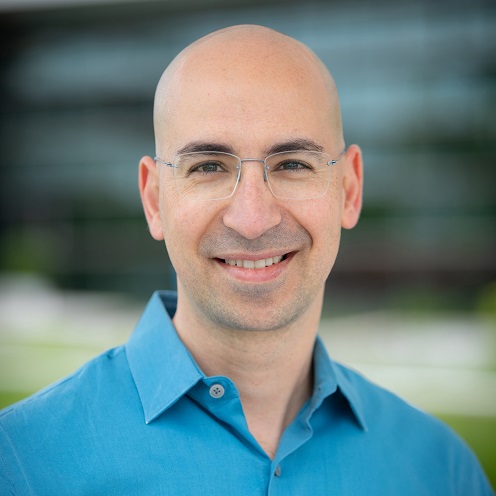
Highlights
Recent Talks
Venue
ECAI-23 (keynote), October 2023
Abstract
Sortition is a storied paradigm of democracy built on the idea of choosing representatives through lotteries instead of elections. In recent years this idea has found renewed popularity in the form of citizens’ assemblies, which bring together randomly selected people from all walks of life to discuss key questions and deliver policy recommendations. A principled approach to sortition, however, must resolve the tension between two competing requirements: that the demographic composition of citizens’ assemblies reflect the general population and that every person be given a fair chance (literally) to participate. I will describe our work on designing, analyzing and implementing randomized participant selection algorithms that balance these two requirements. I will also discuss practical challenges in sortition based on experience with the adoption and deployment of our open-source system, Panelot.
Venue
MD4SG Workshop, June 2019
Abstract
I will present the 'virtual democracy' framework for the design of ethical AI. In a nutshell, the framework consists of three steps: first, collect preferences from voters on example dilemmas; second, learn models of their preferences, which generalize to any (previously unseen) dilemma; and third, at runtime, predict the voters' preferences on the current dilemma, and aggregate these virtual 'votes' using a voting rule to reach a decision. I will focus on two instantiations of this approach: a proof-of concept system that decides ethical dilemmas potentially faced by autonomous vehicles, and a decision support tool designed to help a Pittsburgh-based nonprofit allocate food donations to recipient organizations. These projects bridge AI, social choice theory, statistics, and human-computer interaction; I will discuss challenges in all of these areas.
Venue
University of Washington CS Colloquium, November 2017
Abstract
Computational social choice deals with algorithms for aggregating individual preferences or opinions towards collective decisions. AI researchers (including myself) have long argued that such algorithms could play a crucial role in the design and implementation of multiagent systems. However, in the last few years I have come to realize that the "killer app" of computational social choice is helping people — not software agents — make joint decisions. I will illustrate this theme through two recent endeavors: Spliddit.org, a website that offers provably fair solutions to everyday problems; and Robovote.org, which provides optimization-driven voting methods. Throughout the talk, I will devote special attention to the theoretical foundations and results that make these services possible.
Daniel Halpern to join the University of Toronto
Congratulations to Daniel Halpern, who has accepted a position as assistant professor of computer science at the University of Toronto! He will spend some time as a research scientist at Google Research before starting his faculty position.
Jamie Tucker-Foltz to join Yale
Congratulations to Jamie Tucker-Foltz, who has accepted a position as assistant professor of operations and computer science at Yale! He will start the position in July.
Interview in Science News Explores
Science News Explores, a science magazine for kids, published a cute interview with me. Their definition of “solution” (under “power words”) is not quite what I had in mind, though.
Daniel Halpern wins a Siebel Scholarship
Congratulations to Daniel Halpern, who was selected as a 2024 Siebel Scholar.
SIGecom Mid-Career Award
I was selected to receive the SIGecom Mid-Career Award for “breakthroughs in theory and practice of democracy and social choice, and contributions to SIGecom.” I look forward to giving the award talk at EC’24.
Spliddit is back
Our not-for-profit fair division website, spliddit.org, died in 2022 at the ripe old age of 8. It’s now miraculously working again thanks to a heroic effort by Nisarg Shah and Soroush Ebadian. The resurrected version has a vastly improved algorithm for dividing chores!
Bailey Flanigan to Join MIT
Congratulations to Bailey Flanigan, who has accepted a position as assistant professor of political science and EECS at MIT! She will join MIT in 2025.
Greg Kehne to join WashU
Congratulations to Greg Kehne, who has accepted a tenure-track faculty position in the Department of Computer Science and Engineering at Washington University at St. Louis! He will join WashU in summer 2024.
AAAI Fellow
I was elected as Fellow of the Association for the Advancement of AI (AAAI), for “contributions to AI and society, including foundational work on economic paradigms and practical impact on governance and group decisions.”
Kalai Prize in Game Theory and Computer Science
Our paper “The Unreasonable Fairness of Nash Welfare” won the Game Theory Society’s Prize in Game Theory and Computer Science in honor of Ehud Kalai, awarded to one paper every four years. The prize talk will be given by Nisarg Shah at the 7th World Congress of the Game Theory Society.
Op-ed about the Israeli constitutional crisis in the Washington Post
An op-ed that I wrote with Liav Orgad was published today in the Washington Post. Israel needs a constitution, but who can be trusted to write it? Our answer is a bunch of random people — that is, a citizens’ assembly.
Jamie Tucker-Foltz wins a Google PhD Fellowship
Congratulations to Jamie Tucker-Foltz, who was selected as a Google PhD Fellow in Algorithms, Optimization and Markets (with a secondary area in Algorithmic Fairness).
EC-23 Exemplary Paper in the Applied Modeling Track
Our paper “Welfare-Maximizing Pooled Testing” has been selected as the exemplary paper in the applied modeling track of EC-23. The paper is joint work with Simon Finster, Michelle González Amador, Edwin Lock, Francisco Marmolejo-Cossío, and Evi Micha.
Abstract:
In an epidemic, how should an organization with limited testing resources safely return to in-person activities after a period of lockdown? We study this question in a setting where the population at hand is heterogeneous in both utility for in-person activities and probability of infection. In such a period of re-integration, tests can be used as a certificate of non-infection, whereby those in negative tests are permitted to return to in-person activities for a designated amount of time. Under the assumption that samples can be pooled, the question of how to allocate a limited testing budget in the population to maximize the aggregate utility (i.e. welfare) of negatively-tested individuals who return to in-person activities is non-trivial, with a large space of potential testing allocations.
We show that non-overlapping testing allocations, which are both conceptually and (crucially) logistically more simple to implement, are approximately optimal, and we design an efficient greedy algorithm for finding non-overlapping testing allocations with approximately optimal welfare. In computational experiments, we highlight the efficacy and viability of our greedy algorithm in practice. To the best of our knowledge, we are also first to implement and provide causal evidence on the benefits of utility-weighted pooled testing in a real-world setting. Surprisingly, our pilot study at a higher education research institute in Mexico finds no evidence that performance and mental health outcomes of participants in our testing regime are worse than under the first-best counterfactual of full reopening without testing.
Paul Gölz to join Cornell University
Congratulations to Paul Gölz, who has accepted a tenure-track faculty position in the Department of Operations Research and Information Engineering at Cornell University! He will join Cornell in summer 2024.
Feature article in Scientific American
A feature article that I wrote for Scientific American, “A More Perfect Algorithm,” was published in the November 2022 issue; the online version is available here (and the print PDF is available by request). The article describes our work on algorithms for selecting citizens’ assemblies, which was led by Bailey Flanigan, Paul Gölz, and Gili Rusak.
Greg Kehne wins a Siebel Scholarship
Congratulations to Greg Kehne for winning a 2023 Siebel Scholarship.
AIJ Prominent Paper Award
My paper “Optimal social choice functions: A utilitarian view,” co-authored with Craig Boutilier, Ioannis Caragiannis, Simi Haber, Tyler Lu, and Or Sheffet, is the winner of the of the 2022 AIJ Prominent Paper Award, which “recognizes outstanding papers published not more than 7 years ago in the AI Journal that are exceptional in their significance and impact.”
Anson Kahng to join the University of Rochester
Congratulations to Anson Kahng, who has accepted a tenure-track faculty position in the Department of Computer Science and the Goergen Institute of Data Science at the University of Rochester! He will join Rochester in fall 2022, after spending a year as a postdoc at the University of Toronto.
Work on sortition published in Nature
Our paper “Fair Algorithms for Selecting Citizens’ Assemblies” was published earlier today in Nature. See also the Harvard news story and commentary by Mark Warren. The work was led by Bailey Flanigan and Paul Gölz, in collaboration with Anupam Gupta and Brett Hennig.
Panelot is online
Panelot (panel selection by lot) is our new not-for-profit service that helps organizations select citizens’ panels at random — a democratic paradigm known as sortition. For more details, see the website’s about page.
Paul wins a JP Morgan AI Research Fellowship
Congratulations to Paul Gölz for winning a 2021 JP Morgan AI Research Fellowship.
Daniel and Jamie win NSF Fellowships
Congratulations to Daniel Halpern and Jamie Tucker-Foltz for winning NSF Graduate Research Fellowships.
Op-ed about social media in the Washington Post
My op-ed “Social media may have contributed to record turnout in the 2020 election” was published today in the Washington Post. (I would normally elaborate on what it’s about but the excruciatingly long headline says it all.)
New course: Optimized Democracy
In Spring 2021 I’ll be teaching a new graduate course, Optimized Democracy (CS 238), at Harvard. It will examine the mathematical and algorithmic foundations of democracy, running the gamut from theory to applications. The goal is to provide students with a rigorous perspective on, and a technical toolbox for, the design of better democratic systems. Topics include computational social choice (identifying optimal voting rules), fair division with applications to political redistricting (avoiding gerrymandering) and apportionment (allocating seats on a representative body), sortition (randomly selecting citizens’ assemblies), liquid democracy (transitively delegating votes), and weighted voting games (analyzing legislative power through cooperative game theory).
IFAAMAS Influential Paper Award
My paper “Approximate mechanism design without money,” co-authored with Moshe Tennenholtz, is co-winner of the 2020 IFAAMAS Influential Paper Award. The citation reads:
This paper was the first to formally initiate the field of approximate mechanism design without money, as the title accurately suggests. It blends a key contribution from economics (mechanism design without money) and a key contribution from computer science (approximation algorithms), thus bonding the two disciplines further. Its publication has led to an explosion of papers on mechanism design without money. The concept has been applied to a vast number of fundamental problems such as facility location, resource allocation/cake-cutting, scheduling, assignment problem, matching/kidney exchange, voting, classification, auctions without money, and automated mechanism design. The extended version of the paper, appearing in the ACM Transactions of Economics and Computation in 2013, received the distinction of the ACM Computing Reviews “Best of 2013”.
Op-ed about AI and games in the Washington Post
My op-ed “It’s time for AI to outgrow gaming” was published today in the Washington Post. I make the case that AI’s success in recreational games should be harnessed for real-world strategic interactions.
New position at Harvard
I’m excited to announce that I’ll join Harvard University as Gordon McKay Professor of Computer Science on January 1, 2020.
I’m so grateful to my collaborators, friends, colleagues, students, and mentors at CMU for 8 perfect years.
Social Choice and Welfare Prize
I was selected to receive the 2020 Social Choice and Welfare Prize, together with Pietro Ortoleva of Princeton University. The prize is given by the Society for Social Choice and Welfare every two years to one or two researchers who are at most 40 years old. I will deliver the prize lecture at the 15th Meeting of the Society for Social Choice and Welfare, which will take place in June 2020 in Mexico City.
Alex Psomas to join Purdue University
Congratulations to Alex Psomas for accepting a faculty position at Purdue University! He will join the Department of Computer Science at Purdue as Assistant Professor in fall 2020. Alex received his PhD in computer science from UC Berkeley (where he was advised by Christos Papadimitriou) in 2017, and subsequently joined my group as a postdoc.
Tom Yan wins an NSF Fellowship
Congratulations to Tom Yan for winning a 2019-2022 National Science Foundation Graduate Research Fellowship.
EC-20 PC co-chair
Together with Michael Ostrovsky, I’m serving as co-chair of the program committee of the 21st ACM Conference on Economics and Computation (EC-20).
Gerdus Benade to join Boston University
Congratulations to Gerdus Benade for accepting a faculty position at Boston University! He will join the Questrom School of Business at BU as Assistant Professor of Information Systems in fall 2019. Gerdus has been a PhD student in operations research since 2014. His thesis committee is co-chaired by John Hooker and myself.
Bloomberg Opinion
My piece about the “tragedy of the commons” playing out in AI research appeared today in Bloomberg Opinion. The plan is that I’ll write for them on a regular basis, so stay tuned for more.
Nika Haghtalab wins the SCS Distinguished Dissertation Award
My former PhD student Nika Haghtalab (co-advised with Avrim Blum) won the SCS Distinguished Dissertation Award, given annually for the best PhD thesis in the School of Computer Science. Her thesis is entitled “Foundation of Machine Learning, by the People, for the People.”
AAAI Council
I was elected to a 3-year term on the Executive Council of the Association for the Advancement of Artificial Intelligence (AAAI).
Nika Haghtalab to join Cornell University
Congratulations to Nika Haghtalab for accepting a faculty position at Cornell University! She will join Cornell as Assistant Professor of Computer Science in fall 2019. Nika has been a PhD student in the Computer Science Department since 2013, and is co-advised by Avrim Blum and myself.
ICAPS-18 best paper
Our paper “The Provable Virtue of Laziness in Motion Planning” has been selected to receive the best paper award at the 28th International Conference on Automated Planning and Scheduling, out of 209 submissions. The paper is joint work with Nika Haghtalab (CMU), Simon Mackenzie (CMU), Oren Salzman (CMU), and Sidd Srinivasa (UW).
Abstract: The Lazy Shortest Path (LazySP) class consists of motion-planning algorithms that only evaluate edges along shortest paths between the source and target. These algorithms were designed to minimize the number of edge evaluations in settings where edge evaluation dominates the running time of the algorithm; but how close to optimal are LazySP algorithms in terms of this objective? Our main result is an analytical upper bound, in a probabilistic model, on the number of edge evaluations required by LazySP algorithms; a matching lower bound shows that these algorithms are asymptotically optimal in the worst case.
Guggenheim Fellowship
I am honored to have been named a Guggenheim fellow, together with 172 other scholars, artists, and scientists.
Op-ed about redistricting in the Washington Post
An op-ed that I wrote with Wes Pegden was published by the Washington Post. We discuss our cake-cutting-inspired redistricting protocol, which we presented and analyzed in our paper with Dingli Yu.
Recent press: solution for gerrymandering
In a new paper with Wes Pegden and Dingli Yu, we propose a political redistricting protocol with provable guarantees. This work was covered by the Pittsburgh Post-Gazette, New Scientist, Axios, and the Wisconsin Public Radio.
Update: Wes and I gave a live interview on WDET’s Detroit Today with Stephen Henderson. It’s available here, from 24:51 until 35:25.
Update: New story in Slate.
Recent press: ethical decision making
Our new paper, A Voting-Based System for Ethical Decision Making, is the topic of a nice story by Jon Christian in The Outline (which led to an especially lively discussion on Reddit). Pradeep Ravikumar also gave a great interview about this project on the radio show AirTalk.
Update: See also the Axios story and the Bloomberg opinion piece.
New Scientist story on fair rent division
New Scientist published a story about our EC’16 rent division paper, which will also be presented at the IJCAI’17 Sister Conference Track. (The story was subsequently picked up by the UK tabloid Metro.)
Misc. recent press: game theory, cake cutting, and online dating
New Scientist just published a nice story about our IJCAI’17 paper with the catchy title “Why You Should Charge Your Friends for Borrowing Your Stuff” (short answer: because in a game where non-rivalrous goods are bought and shared on a network, equilibria are more efficient, in theory and experiments, when access costs are imposed).
Here are some other random topics I’ve weighed in on recently (not covered by other news):
* Aziz and Mackenzie’s breakthrough result on bounded envy-free cake cutting, in the Sydney Morning Herald and Quanta Magazine.
* AI and online dating, in the Boston Globe.
Swaprava Nath to join IIT Kanpur
Congratulations to Swaprava Nath, who will join the Indian Institute of Technology, Kanpur, as Assistant Professor in July. I’ve hosted Swaprava as a postdoc since 2015; he is supported by the Fulbright-Nehru postdoctoral fellowship.
Nisarg Shah wins the Victor Lesser Distinguished Dissertation Award
My former PhD student Nisarg Shah won the Victor Lesser Distinguished Dissertation Award, given annually for the best thesis in the area of autonomous agents and multiagent systems. His thesis is entitled “Optimal Social Decision Making.” He will present his thesis research in a plenary talk at AAMAS-17 in Brazil.
Op-ed in Wired
An opinion piece that I wrote was published today in Wired. It’s about computational social choice (although I don’t call it that) and a bit of politics.
Pittsburgh Post-Gazette interview
I was interviewed by Tim Grant from the Pittsburgh Post-Gazette about RoboVote and voting more generally. Worth checking out, if only for the bizarre portrait photo with the hovering phones!
RoboVote: Public launch and press coverage
RoboVote (http://robovote.org), a not-for-profit voting website that provides optimization-driven methods for making collective decisions, has launched today! Check out RoboVote’s about page for (somewhat AI-centric) information about the website, its algorithms, and the theory underlying them, as well as info about the amazing team.
Press coverage (newest to oldest): Wired, Pittsburgh Post-Gazette, ExtremeTech, Daily Mail, Digital Trends, Marketplace, CMU homepage
EC-16 best paper
Our paper “Which Is the Fairest (Rent Division) of Them All” has been selected to receive the best paper award at the 17th ACM Conference on Economics and Computation, out of 242 submissions. The paper is joint work with Kobi Gal and Moshik Mash of Ben Gurion University, and my postdoc Yair Zick.
Abstract: What is a fair way to assign rooms to several housemates, and divide the rent between them? This is not just a theoretical question: many people have used the Spliddit website to obtain envy-free solutions to rent division instances. But envy freeness, in and of itself, is insufficient to guarantee outcomes that people view as intuitive and acceptable. We therefore focus on solutions that optimize a criterion of social justice, subject to the envy freeness constraint, in order to pinpoint the “fairest” solutions. We develop a general algorithmic framework that enables the computation of such solutions in polynomial time. We then study the relations between natural optimization objectives, and identify the maximin solution, which maximizes the minimum utility subject to envy freeness, as the most attractive. We demonstrate, in theory and using experiments on real data from Spliddit, that the maximin solution gives rise to significant gains in terms of our optimization objectives. Finally, a user study with Spliddit users as subjects demonstrates that people find the maximin solution to be significantly fairer than arbitrary envy-free solutions; this user study is unprecedented in that it asks people about their real-world rent division instances. Based on these results, the maximin solution has been deployed on Spliddit since April 2015.
Nisarg Shah to join the University of Toronto
Congratulations to Nisarg Shah, who has accepted a tenure-track faculty position in the Department of Computer Science at the University of Toronto! He will join U of T in fall 2017, after spending a year as a postdoc at Harvard’s Center for Research in Computation and Society. I have had the privilege of advising Nisarg since he joined our PhD program in fall 2011 (which is the same time I myself joined CMU); he is defending on June 28.
Handbook of Computational Social Choice
The Handbook of Computational Social Choice, which I have co-edited together with Felix Brandt, Vince Conitzer, Ulle Endriss, and Jerome Lang, has been officially published.
Description: The rapidly growing field of computational social choice, at the intersection of computer science and economics, deals with the computational aspects of collective decision making. This handbook, written by thirty-six prominent members of the computational social choice community, covers the field comprehensively. Chapters devoted to each of the field’s major themes offer detailed introductions. Topics include voting theory (such as the computational complexity of winner determination and manipulation in elections), fair allocation (such as algorithms for dividing divisible and indivisible goods), coalition formation (such as matching and hedonic games), and many more. Graduate students, researchers, and professionals in computer science, economics, mathematics, political science, and philosophy will benefit from this accessible and self-contained book.
A PDF of the book is freely available online. To open the document, please enter the password cam1CSC (strangely enough, this password can be disseminated legally, so you are encouraged to shout it from the rooftops). The link is here:
http://www.cambridge.org/
Alternatively, the book can be purchased through the Cambridge University Press website (http://www.cambridge.org/
Yair Zick to join the National University of Singapore
Congratulations to Yair Zick for accepting a faculty position at the National University of Singapore! He will join NUS as Assistant Professor of Computer Science in the summer. Yair has been a postdoc at CMU since April 2014, jointly hosted by Anupam Datta and me. Before that he completed his PhD at Nanyang Technological University, Singapore, where he worked with Edith Elkind.
Nika Haghtalab wins an MSR PhD fellowship
Congratulations to Nika Haghtalab for winning a 2016-2018 Microsoft Research PhD fellowship. Nika is co-advised by Avrim Blum and me.
Dagstuhl workshop on fair division
Together with Yonathan Aumann, Steven Brams, and Jérôme Lang, I am organizing a Dagstuhl workshop on Fair Division. The workshop will take place on June 6-10, 2016.
Interview in New Scientist
New Scientist published a “one minute interview” with me about AI and fair division.
IJCAI Computers and Thought Award
I received the IJCAI Computers and Thought Award at IJCAI’15 in Buenos Aires. A narrated video of my presentation at the conference is now available online.
Spliddit: New apps and press coverage
A new version of Spliddit, featuring two new apps and improvements to the old ones, has officially launched!
Press coverage (newest to oldest): Metro, New Scientist #2, Quanta Magazine, The New York Times #2, New Scientist #1, Pittsburgh Magazine, GeekWire, NSF podcast, Fast Company, Pittsburgh Post-Gazette, Lifehacker, Slashdot, Gizmodo, Nautilus Magazine, The New York Times #1.
Nika Haghtalab wins an IBM PhD fellowship
Congratulations to Nika Haghtalab for winning a 2015-2016 IBM PhD fellowship, with a statement entitled “Learning Theory for Societal Good”. Nika is co-advised by Avrim Blum and me.
Sloan Research Fellowship
I was named a Sloan Research Fellow, together with 125 other young researchers in chemistry, computer science, economics, mathematics, computational and evolutionary molecular biology, neuroscience, ocean sciences, and physics.
EC-14 best student paper
Congratulations to Junxing Wang for winning the best student paper award at EC-14, for our joint paper “Fair Enough: Guaranteeing Approximate Maximin Shares”.
Nisarg Shah wins a Facebook PhD fellowship
Congratulations to Nisarg Shah for winning a 2014-2015 Facebook PhD fellowship, with a statement entitled “Economic Foundations of Social Computing”. There are 11 winners overall, 3 of whom are from CMU!